In 2016, an AI program called AlphaGo made headlines by defeating one of the world’s top Go players, Lee Sedol, winning four games of a five-game match. AlphaGo learned the strategy board game by studying the techniques of human players, and by playing against versions of itself. While AI systems have long been learning from humans, scientists are now asking if the learning could go both ways. Can we learn from AI?
Karina Vold, an assistant professor at U of T’s Institute for the History and Philosophy of Science and Technology, believes we can. She is studying how humans can learn from technologies such as the neural networks that underlie today’s AI systems.
“In the case of Go, professional players learn through proverbs such as ‘line two is the route to defeat,’ or ‘always play high, not low,’” says Vold, who works at the intersection of philosophy and cognitive science, and is affiliated with the Schwartz Reisman Institute for Technology and Society. Those proverbs can be useful, but they can also be limiting, impeding a player’s flexibility. AlphaGo, meanwhile, gleans insights – a term that Vold believes is appropriate – from digesting enormous volumes of data. “Because AlphaGo learns so differently, it did moves that were considered very unlikely for a good human player to make,” Vold says.
A key moment occurred in the second game, on the 37th move, when AlphaGo played a move that took everyone – including Sedol – by complete surprise. As the game went on, however, move 37 proved to be a masterstroke. Human Go players “are now studying some of the moves that AlphaGo made and trying to come up with new sorts of proverbs and new ways of approaching the game,” says Vold.
Vold believes the possibility of humans learning from AI extends beyond game playing. She points to AlphaFold, an AI system unveiled by DeepMind (the same company behind AlphaGo) in 2018, that predicts the effects of proteins based on their structure. Proteins are made up of sequences of amino acids, which can fold and form complex 3D structures. The protein’s shape determines its properties, which in turn determine its potential efficacy in new drugs to treat diseases. Because proteins can fold in millions of different ways, however, it is impossible for human researchers to work through all the combinations. “This was a long-standing grand challenge in biology that had been unsolved,” says Vold, but in which AlphaFold “was able to make great advances.”
Even in cases where humans may have to rely on the sheer computing power of an AI system to tackle certain problems – as with protein folding – Vold believes artificial intelligence can guide human thinking by reducing the number of paths or conjectures that are worth pursuing. While humans may not be able to duplicate the insights an AI model makes, it is possible “that we can use these AI-driven insights as scaffolding for our own cognitive pursuits and discoveries.”
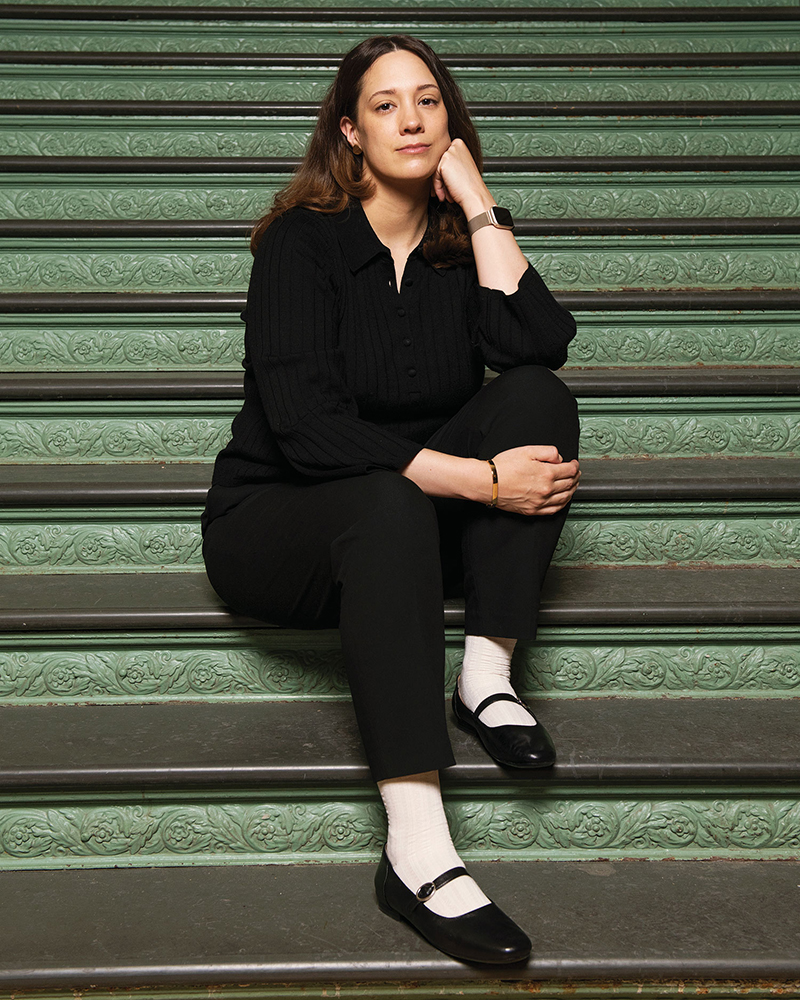
In some cases, Vold says, we may have to rely on the “AI scaffolding” permanently, because of the limitations of the human brain. For example, a doctor can’t learn to scan medical images the same way that an AI processes the data from such an image; the brain and the AI are just too different. But in other cases, an AI’s outputs “might serve as cognitive strategies that humans can internalize [and, in so doing, remove the ‘scaffolding’],” she says. “This is what I am hoping to uncover.”
Vold’s research also highlights the issue of AI “explainability.” Ever since AI systems started making headlines, concerns have been raised over their seemingly opaque workings. These systems, and the neural networks they employ, have often been described as “black boxes.” We may be impressed by how quickly they appear to solve certain kinds of problems, but it might be impossible to know how they arrived at a particular solution.
Vold believes it may not always be necessary to understand exactly how an AI system does what it does in order to learn from it. She notes that the Go players who are now training on the moves that AlphaGo made don’t have any inside information from the system’s programmers as to why the AI made the moves it did. “Still, they are learning from the outputs and incorporating the moves into their own strategic considerations and training. So, I believe that at least in some cases, AI systems can function like black boxes, and this will be no hindrance to our learning from them.”
Yet there may still be situations where we won’t be satisfied unless we can see inside the black box, so to speak. “In other cases, we may need to understand how the system works to really learn from it,” she says. Trying to distinguish cases where explainability is crucial from those where a black box model is sufficient “is something I’m still thinking about in my research,” says Vold.
This article was published as part of our series on AI. For more stories, please visit AI Everywhere.
Recent Posts
U of T’s Feminist Sports Club Is Here to Bend the Rules
The group invites non-athletes to try their hand at games like dodgeball and basketball in a fun – and distinctly supportive – atmosphere
From Mental Health Studies to Michelin Guide
U of T Scarborough alum Ambica Jain’s unexpected path to restaurant success
A Blueprint for Global Prosperity
Researchers across U of T are banding together to help the United Nations meet its 17 sustainable development goals